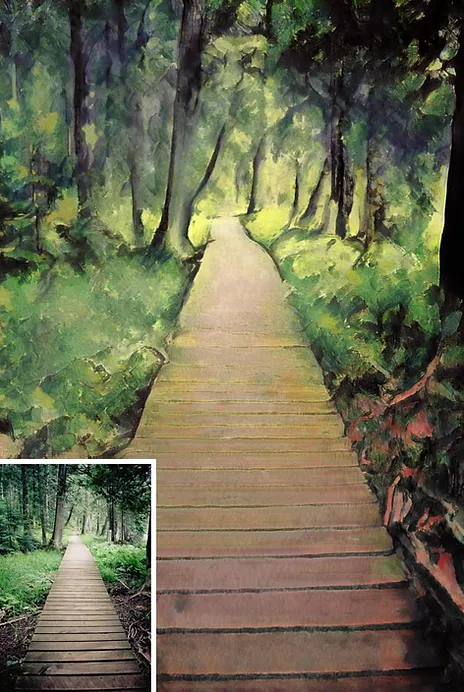
Neural Style Transfer
In recent years, the transfer of artistic style to real photos has received much attention. While much of this research has aimed at speeding up processing, the approaches are still lacking from a principled, art historical standpoint. The Computer Vision Group has addressed these shortcomings in several papers and developed models for artistic style transfer.
The following aspects have been addressed in the publications:
(1) A style is more than just a single image, but previous work is limited to only a single instance of a style and shows no benefit from more images. Our approach utilizes a collection of style images from an artist to represent the style in its fullness.
See: Sanakoyeu et al., ECCV, 2018.
(2) Moreover, previous work has relied on a direct comparison of art in the domain of RGB images or on CNNs pre-trained on ImageNet, which requires millions of labelled object bounding boxes and can introduce an extra bias, since it has been assembled without artistic consideration. To circumvent these issues, we propose a style-aware content loss, which is trained jointly with a deep encoder-decoder network for real-time, high-resolution stylization of images and videos.
See: Sanakoyeu et al., ECCV, 2018.
(3) The explicit transformation of image content has been mostly neglected. While artistic style affects formal characteristics of an image, such as color, shape or texture, it also deforms, adds or removes content details. We explicitly focus on a content-and style-aware stylization of the respective content image.
See: Kotovenko et al. A Content Transformation, CVPR, 2019
(4) Artists rarely paint in a single style throughout their career. More often they change styles or develop variations of it. In addition, artworks of different styles and even within one style depict real content differently. We present a novel approach which captures particularities of a style, regards subtle changes and separates style and content.
See: Kotovenko et al. Content and Style Disentanglement, ICCV, 2019.
Our models have produced compelling stylizations displaying defining formal properties of artistic styles. In addition, the robustness and speed of our models enable a video stylization in real-time and high definition. A link to our video stylizations can be found HERE.
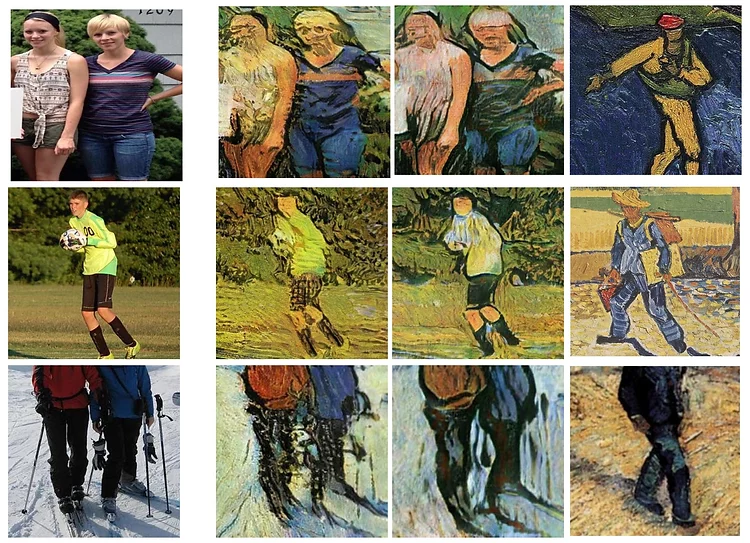
The figure illustrates the impact of the content transformation block presented in Kotovenko et al. A Content Transformation, CVPR, 2019. The first column displays the real photo, the second the result without and the third column with using the content transformation block. We see that our model was able to alter persons in a manner appropriate to van Gogh’s style.
The last column provides details from the artist’s paintings to highlight the validity of our approach.
Image source: Kotovenko et al. A Content Transformation, CVPR, 2019.
Selected Publications
2022
ArtFID: Quantitative Evaluation of Neural Style Transfer Conference
Proceedings of the German Conference on Pattern Recognition (GCPR '22) (Oral), 2022.
Text-Guided Synthesis of Artistic Images with Retrieval-Augmented Diffusion Models Conference
Proceedings of the European Conference on Computer Vision (ECCV) Workshop on Visart, 2022.
2021
Rethinking Style Transfer: From Pixels to Parameterized Brushstrokes Conference
Proceedings of the IEEE Conference on Computer Vision and Pattern Recognition (CVPR), 2021.
2019
Using a Transformation Content Block For Image Style Transfer Conference
Proceedings of the IEEE Conference on Computer Vision and Pattern Recognition (CVPR), 2019.
Content and Style Disentanglement for Artistic Style Transfer Conference
Proceedings of the Intl. Conf. on Computer Vision (ICCV), 2019.
2018
A Style-Aware Content Loss for Real-time HD Style Transfer Conference
Proceedings of the European Conference on Computer Vision (ECCV) (Oral), 2018.
Stylized images in the styles of Vincent van Gogh (1853-1890) (left) and Berthe Morisot (1841-1895) (right). The real content photos can be seen in the bottom left of each image. Image sources: Kotovenko et al. A Content Transformation, CVPR, 2019.
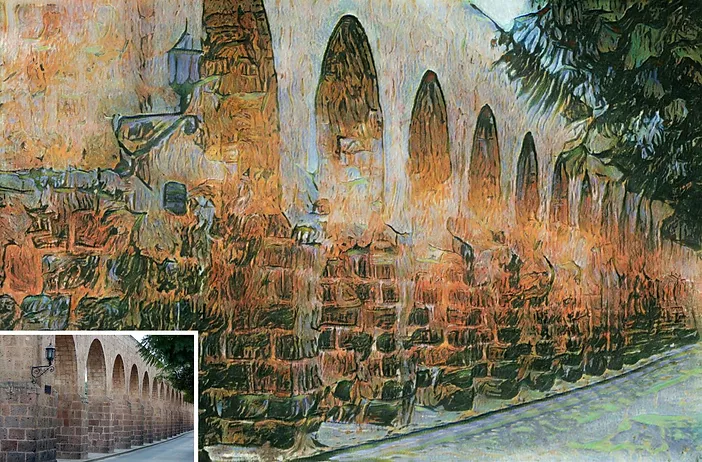
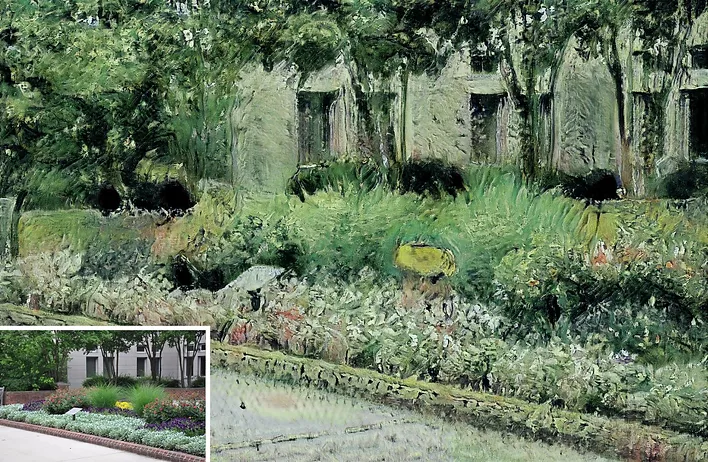